Blog > Data Science and AI > Applications of Machine Learning
Applications of Machine Learning (Examples and Use Cases)
Introduction
Embarking on an exploration of Machine Learning (ML) unveils a world where innovation and intelligence converge to redefine the boundaries of what technology can achieve. Positioned at the forefront of the ongoing artificial intelligence (AI) revolution, ML represents not just a mere component but a driving force that’s actively reshaping how we interact with the world around us. It’s a catalyst for change, transforming data into insights, insights into action, and action into advancement across many fields. From revolutionising healthcare with predictive diagnostics to reshaping the finance sector with automated trading algorithms, ML’s influence is pervasive and profound.
Understanding Machine Learning
At its core, machine learning embodies the art and science of enabling computers to learn from and interpret data, making informed decisions without needing explicit instructions for every scenario. This capability hinges on a diverse array of algorithms and models, each designed to tackle different types of learning and problem-solving tasks within the vast landscape of AI. There are three fundamental types of learning methodologies that dictate how models interact with data and evolve over time.
- Supervised Learning: Represents an approach where models are trained on a labelled dataset to predict outcomes and recognise patterns. Common applications range from spam detection in emails to predicting customer behaviour.
- Unsupervised Learning: This approach dives into datasets that lack explicit labels. Here, the magic lies in the model’s ability to discern structure, patterns, and correlations without any human supervision or explicit instructions. This type of learning is useful in market segmentation, anomaly detection, and discovering novel scientific phenomena.
- Reinforced Learning: Represents a dynamic and interactive form of learning where an agent learns to make decisions by performing actions and receiving feedback in the form of rewards or penalties—much like how humans learn from their environment. It’s this technique that underpins the development of sophisticated game-playing AI, like online chess or Go, and autonomous vehicles that learn to navigate complex environments.
These foundational learning strategies empower ML to transcend traditional data analysis boundaries, transforming it into a potent tool for predictive analytics and autonomous decision-making. Through the intricate dance of algorithms and data, ML is forging a future where machines not only mimic cognitive functions but also uncover insights that drive innovation and solve complex challenges across various domains.
What Can Machine Learning Be Used For
Machine learning is widely used across numerous everyday applications, automating and enhancing processes in various sectors. For instance, consumers are able to leverage both the chatbot and recommendation engine to streamline their interactions and personalise their experiences. Chatbots provide immediate, accurate responses to inquiries, learning from each interaction to enhance future communications. This improves customer service efficiency and satisfaction. Meanwhile, recommendation engines analyse past behaviour and preferences to suggest products, services, or content, significantly boosting user engagement and satisfaction by making interactions more relevant and tailored to individual needs.
Additionally, ML plays a critical role in fraud and cyberthreat detection. By continuously learning from transactional data and user behaviour, ML models can identify what may potentially be fraudulent or potential security breaches by analysing patterns and anomalies. This capability is crucial for financial institutions, e-commerce platforms, and cybersecurity firms, providing them with the tools to protect their operations and their customers’ data effectively.
These versatile applications underscore machine learning’s role in not only streamlining operations but also in driving innovations that enhance user experiences and safeguard vital business and customer data.
Applications of Machine Learning Across Industries
Applications of machine learning are vast and varied, demonstrating its versatility in tackling complex problems and enhancing user engagement across different platforms.
Social Media
When it comes to social media, machine learning has become a transformative force, enhancing user experiences through advanced personalisation techniques. Personalised content delivery makes use of machine learning algorithms to analyse users’ interaction patterns, preferences, and engagement history, enabling platforms to curate news feeds and recommend content that aligns perfectly with individual tastes. Tiktok’s success, for instance, could be attributed to the stickiness of its recommendations that are powered by machine learning models.
Facial recognition and auto-tagging technologies, powered by machine learning, further augment this experience by accurately identifying and tagging individuals in photos. These systems, adept at analysing extensive datasets of facial features, streamline the process of tagging, making it easier for users to share memories with friends and family. This capability not only simplifies photo management but also enriches social connections by effortlessly sharing moments and memories, fostering a more connected and engaged user community.
Additionally, sentiment analysis through ML offers invaluable insights into public perception of brands and products on social media. By evaluating the tone and context of user comments and posts, algorithms can assess overall sentiment, providing businesses with critical feedback for managing their online reputation and responding effectively to consumer opinions.
E-commerce and Retail
The e-commerce and retail sectors have witnessed a revolution with the integration of machine learning, particularly through product recommendations tailored to individual behaviours and preferences. Recommendation systems utilise insights from user data, including purchase history and browsing patterns, to curate personalised product suggestions. This targeted approach markedly improves the shopping experience, leading to increased sales by catering directly to individual preferences.
Additionally, the shopping experience is further enriched through enhanced customer service, facilitated by introducing chatbots and virtual assistants. These tools, powered by machine learning, provide prompt, efficient, and personalised assistance to shoppers, seamlessly addressing inquiries and improving overall customer satisfaction.
Meanwhile, in inventory management, ML employs predictive analytics to anticipate demand fluctuations, enabling efficient stock level optimisation. This foresight prevents overstocking or stockouts, ensuring that consumer demand is met without surplus inventory, thereby optimising operational costs.
Healthcare
Machine learning’s impact on healthcare is profound, particularly in disease diagnosis and prediction. ML models process and learn from medical imaging and patient data to accurately predict diseases and medical conditions, facilitating early diagnosis and treatment. This early intervention capability is crucial for effective disease management and improving patient outcomes.
This is further exemplified in the transformative approach of personalised medicine, where machine learning is used to tailor healthcare treatments to individuals’ unique genetic profiles, lifestyles, and health histories. This bespoke method of delivering healthcare not only promises to enhance the effectiveness of treatments but also to minimise side effects and unlock new pathways for healthcare.
Beyond enhanced patient care, ML has also accelerated the identification of new drug candidates by analysing biological data and chemical compounds. This process, which traditionally takes years and significant financial investment, is made more efficient through machine learning-driven simulations, paving the way for faster development of life-saving medications.
Finance and Banking
In the finance and banking sector, machine learning is a pivotal innovation, reshaping the way institutions interact with data to safeguard assets, evaluate creditworthiness, and navigate the complexities of the stock market. Fraud detection, for one, has been revolutionised by ML’s anomaly detection techniques, which scrutinise each transaction for patterns deviating from the norm, flagging potential fraud with unprecedented accuracy. This proactive approach to fraud prevention not only protects financial institutions but also secures customer trust.
Similarly, credit scoring has evolved beyond traditional metrics, with ML algorithms analysing vast amounts of financial history data to assess loan eligibility and creditworthiness more accurately. This machine learning-driven analysis offers a nuanced understanding of financial behaviour, enabling more personalised and fair lending decisions.
Another area where machine learning is applied is in algorithmic trading, where machine learning is leveraged to predict stock market movements and devise sophisticated trading strategies. By processing and learning from historical market data, ML models can identify profitable trading opportunities, optimise investment portfolios, and outperform traditional market analysis techniques.
Transportation and Logistics
The transportation and logistics industry is undergoing a significant transformation, propelled by the application of machine learning. Self-driving cars are perhaps the most striking example of this shift, with ML being integral to developing autonomous driving systems. These systems rely on machine learning algorithms to interpret sensor data, allowing vehicles to navigate complex environments safely and efficiently.
Beyond individual vehicles, ML enhances traffic prediction and management, employing real-time data analysis to optimise traffic flow and reduce congestion. By predicting traffic patterns, cities can adjust signal timings and reroute traffic dynamically, improving urban mobility.
Supply chain optimisation further illustrates ML’s impact, utilising predictive models to forecast demand accurately and manage inventory levels. This optimisation ensures supply chains are more resilient, responsive, and efficient, minimising costs while effectively meeting consumer demand.
Speech and Language Processing
ML has dramatically advanced the field of speech and language processing, making interactions with technology more natural and intuitive. Voice-activated assistants, such as Siri, Alexa, and Google Assistant, are powered by ML algorithms that understand and process spoken commands, providing users with information and performing tasks through simple voice prompts. This technology has not only made digital assistants more reliable but also increasingly ubiquitous in daily life.
Machine learning has also made significant strides in language translation, where models like Google’s Neural Machine Translation (GNMT) system are breaking down language barriers and facilitating global communication. These systems analyse the context and nuances of language, offering translations that are remarkably accurate and contextually aware.
Machine learning has also helped businesses through Natural Language Processing (NLP) applications, which allow for the automated monitoring of customer sentiment across social media and review platforms, providing valuable feedback to businesses. It also enables efficient categorisation of text data, streamlining customer service by routing inquiries to the appropriate departments or generating automated responses, thereby enhancing the customer experience.
Image and Video Analysis
Image recognition technology, powered by machine learning, has become indispensable in various fields, including security systems, medical imaging, and social media platforms. In security systems, it aids in identifying potential threats or unauthorised access through facial recognition algorithms. In the healthcare sector, image recognition significantly enhances the accuracy of medical diagnoses by analysing imaging data for signs of diseases, such as tumours, in MRI scans.
Similarly. video surveillance has evolved with machine learning, enabling the real-time analysis of video feeds for security and monitoring purposes. This capability allows for the immediate detection of unusual activities or safety breaches, ensuring prompt response to potential incidents.
When it comes to entertainment, augmented reality (AR) has applied machine learning to its systems, creating immersive user experiences that seamlessly blend virtual elements with the real world. From educational tools that overlay historical facts onto real-world locations to gaming applications that integrate fictional characters into our environment, AR has expanded the ways in which users interact with technology.
Environmental Conservation
In the critical area of wildlife protection, machine learning algorithms play a vital role, enabling the monitoring of endangered species and the prediction of poaching activities. Utilising data from diverse sources such as camera traps and satellite imagery, these algorithms assist conservationists in tracking wildlife, pinpointing areas vulnerable to poaching, and optimising the allocation of protective resources to shield at-risk species.
Similarly, the fight against climate change is strengthened by the capabilities of machine learning. Climate change analysis leverages ML models adept at navigating the complexities of environmental datasets to forecast climatic trends and evaluate potential impacts with heightened accuracy. These models delve into patterns of temperature shifts, rainfall, and other critical environmental indicators to furnish deep insights into how climate change could shape our world. Armed with this knowledge, decision-makers are better equipped to craft informed policies and strategies for climate mitigation, underscoring machine learning’s pivotal role in addressing some of the most pressing environmental challenges of our time.
Challenges and Ethical Considerations
The implementation of machine learning solutions is not without its challenges and ethical considerations. Issues surrounding data privacy are paramount, as machine learning algorithms often require access to vast amounts of personal and sensitive data. Ensuring the ethical use of machine learning and artificial intelligence involves strict adherence to privacy regulations and the safeguarding of data against misuse. Transparency in how ML models make decisions is crucial for building trust and understanding among users, particularly in critical applications, such as healthcare and law enforcement.
The development and deployment of machine learning must prioritise responsible AI practices, emphasising fairness and accountability. This includes addressing biases in data and algorithms to prevent discriminatory outcomes and ensuring that machine learning applications respect ethical standards and societal values. Navigating these challenges responsibly is essential for harnessing the benefits of machine learning while minimising negative impacts, marking a path toward a future where AI and machine learning contribute positively to society.
Future of Machine Learning
The future landscape of machine learning is marked by exciting emerging trends and boundless potential for application across myriad industries. As we peer into the horizon, we envision ML not just as a tool for data analysis but as a fundamental driver of innovation and efficiency.
Future advancements are expected to bring about even more sophisticated algorithms capable of learning from smaller datasets with greater accuracy and less human intervention. This evolution will likely see ML playing a key role in solving some of the world’s most pressing problems, from combating climate change to advancing personalised medicine. Moreover, as ML technology continues to mature, its integration into everyday life and business operations will become even more seamless, opening up new opportunities for automation, predictive analytics, and intelligent systems design
The potential for ML to shape the future of industries such as healthcare, finance, manufacturing, and education is immense, promising not only to enhance operational efficiency but also to drive growth and innovation in ways we are just beginning to imagine.
How Heicoders Academy Can Help
Heicoders Academy stands at the forefront of empowering individuals to navigate and excel in the rapidly evolving landscape of technology, particularly through the AI300 Deploying Machine Learning Systems to the Cloud. This course is meticulously designed to cater to the burgeoning demand for data scientists who also have the skills to deploy machine learning models to production systems – such as AWS cloud.
The curriculum delves into web technologies, modern HTML and CSS, frameworks like FLASK, and tools like GitHub and AWS cloud which are essential for productionizing machine learning models. Overall, the course emphasises on practical application of machine learning in a web environment, preparing students to integrate ML models into web applications effectively.
Parallel to this, the AI200 Applied Machine Learning course offers a deep dive into the world of machine learning, equipping learners with the skills to apply machine learning algorithms across various domains effectively. This course emphasises practical skills in data wrangling, visualisation, and the deployment of machine learning models to solve real-world problems, making it a vital resource for anyone looking to enter or advance in the field of machine learning. By incorporating the practical application of machine learning in web development, Heicoders Academy prepares students to effectively integrate ML models into web applications, enhancing functionality and user experience.
Embrace the Next Wave of Innovation
The wide-reaching influence of machine learning in transforming industries and societal functions underscores its role as a fundamental driver of change, far beyond a mere technological trend. It has revolutionised fields such as healthcare, and has significantly improved the digital experience for users worldwide. The applications of machine learning span a broad spectrum, catalysing operational efficiencies, fostering innovation, and fueling growth across various sectors.
Standing at the brink of this technological revolution, the imperative is clear: to delve deeper into the exploration, learning, and innovative application of machine learning. By embracing the unique challenges and vast opportunities that machine learning presents, we can propel both individuals and organisations to unprecedented levels of achievement, contributing significantly to global progress and enhancing the quality of life. The voyage into the depths of machine learning is only just beginning, heralding an era of boundless potential and transformative impact.
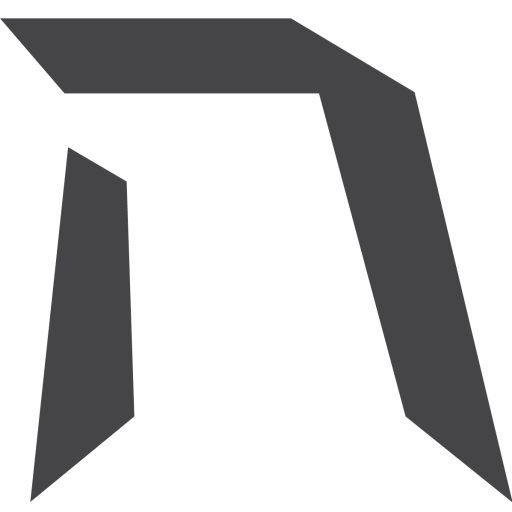