Blog > Data Science & AI / Data Analytics > Navigating Your Career Path in Data Analytics
A Guide to the Most Popular Machine Learning Frameworks and Tools
Introduction to Machine Learning
Machine learning, a branch of artificial intelligence, involves training computers to learn from data, make predictions, and improve their decision-making processes over time without being explicitly programmed. From voice recognition systems and customer service chatbots to predictive data analytics in healthcare and finance, machine learning is changing how we interact with data and technology. Central to this revolution are the languages, libraries, frameworks, and tools designed to streamline the development and deployment of machine learning models.
Most Popular Machine Learning Languages
Programming languages are the foundation upon which software is built, offering a syntax and set of rules for instructing computers to perform various tasks. In the context of machine learning, the choice of programming language can significantly impact the ease of development, model performance, and scalability.
Choosing the right language for a machine learning project involves considering the specific requirements of the project, the language’s performance, and its ecosystem. Python offers a broad range of libraries and ease of use, R provides advanced statistical functions, while Julia offers superior performance for numerical computations.
Python Programming Language
Python is a versatile, high-level programming language known for its ease of learning and readability, making it one of the most popular machine learning languages among beginners and professionals alike. It supports multiple programming paradigms, including procedural, object-oriented, and functional programming, which contributes to its broad application across different fields.
The secret to Python’s dominance in the machine learning sphere lies in its comprehensive library ecosystem. This ecosystem enables practitioners to efficiently handle, process, and transform data, significantly reducing the development time for complex machine learning models. Libraries such as TensorFlow, PyTorch, and Scikit-learn provide foundational code, meaning that engineers can focus on developing solutions rather than building everything from scratch. The simplicity of Python’s syntax and its emphasis on readability foster a collaborative environment where ideas, algorithms, and tools can be easily shared among peers.
R Language
R is designed by statisticians for statisticians, making it a powerhouse for statistical analysis and data visualisation. R’s comprehensive suite of machine learning techniques covers everything from data visualisation to supervised and unsupervised learning, making it a formidable choice for projects that require deep statistical analysis.
R’s application in machine learning is heavily tied to its robust package ecosystem and its built-in support for data analysis and statistical modelling. Libraries such as ‘caret’, ‘mlr’, and ‘randomForest’ offer comprehensive tools that cover a wide range of machine learning techniques, including regression, classification, clustering, and more. R is particularly strong in statistical modelling, making it ideal for projects that require deep statistical analysis and interpretation of complex datasets. The extensive visualisation capabilities of R also make it invaluable for exploratory data analysis, helping to identify underlying patterns or anomalies which inform the development of more sophisticated machine learning models.
Julia Language
Julia is a high-level, dynamic programming language well-suited for numerical and computational science. Its design caters specifically to high-performance numerical analysis and computational science, making it ideal for applications requiring intensive mathematical computations.
Julia is increasingly being adopted in machine learning for its performance in numerical and computational tasks and its ability to handle the extensive computational demands of large-scale machine learning algorithms efficiently. With features like Just-in-Time (JIT) compilation and the ability to directly call C and Fortran libraries without glue code, Julia ensures fast execution, which is crucial for training and deploying large machine learning models. Additionally, Julia’s syntax is specifically suited for mathematical operations, which are a staple in machine learning applications. Its growing ecosystem includes machine learning libraries such as Flux.jl and MLJ.jl, which provide tools and frameworks for creating and testing machine learning models. Julia’s design makes it an ideal choice for applications that require intensive mathematical computations and have high-performance requirements.
Java
Java is a versatile, object-oriented programming language known for its portability across platforms, which is encapsulated in the principle of write once, run anywhere (WORA). It’s widely used in enterprise environments and for building large-scale systems.
In the context of machine learning, Java offers several advantages. Its ease of use, debugging ease, and comprehensive suite of development tools make it a practical choice for developing machine learning applications, especially in large organisations where Java is already a staple. The language’s robust libraries, such as Weka, Deeplearning4j, and MOA, provide powerful tools and frameworks for machine learning, facilitating the development of scalable machine-learning solutions. Additionally, Java’s strong memory management helps in handling large datasets, a common requirement in machine learning applications.
Lisp
Lisp, one of the oldest high-level programming languages, is known for its excellent support for procedural and functional programming with a unique focus on recursion. It’s distinguished by its fully parenthesised prefix notation, which allows for powerful macro systems.
Lisp’s capabilities make it particularly well-suited for rapid prototyping of machine learning algorithms thanks to its flexibility and the dynamic nature of its typing system. Historically, it has been used extensively in research environments, particularly in the development of early artificial intelligence applications. Its symbolic expression and manipulation capabilities offer advantages in developing programs that need to handle symbolic computations, which are common in some areas of machine learning, making it advantageous for tasks involving rule-based inference engines.
C++
C++ is a highly efficient and flexible language that supports both high-level and low-level programming. It combines the ability to make low-level memory manipulations with the features of high-level object-oriented programming.
For machine learning, C++ is particularly valued for its execution speed and use in situations where hardware-level control is necessary. This makes C++ highly suitable for machine learning applications that require real-time execution and resource-efficient computation, such as embedded systems or applications where latency is critical. Libraries like TensorFlow and LightGBM have C++ APIs, allowing developers to integrate machine learning models into applications where performance and speed are critical. Furthermore, C++ supports complex algorithmic challenges and is used in gaming, computer vision, and real-time physical simulations where machine learning models are integral to functionality.
Most Popular Frameworks for Machine Learning
Frameworks in machine learning offer predefined structures and functions to streamline the development of machine learning models, covering tasks from data preprocessing to model evaluation.
Selecting a machine learning framework involves evaluating factors such as the specific needs of the project, the framework’s performance, ease of use, community support, and how well it integrates with other tools and technologies.
Here are some of the most popular machine learning frameworks currently used by practitioners.
Apache Spark MLlib
Apache Spark MLlib is one of the key platforms for big data processing in machine learning. Built atop Apache Spark, an open-source cluster computing framework, Spark MLlib offers advanced APIs optimised for machine learning tasks such as classification, regression, and clustering. The key to Spark MLlib’s success lies in its unmatched ability to handle vast datasets in tandem, enabling parallel processing that ensures both speed and efficiency.
Spark MLlib’s appeal extends beyond its scalability and performance; it is celebrated for its ease of integration with a wide array of data sources and analytical tools. This seamless connectivity allows practitioners to effortlessly combine Spark MLlib with their existing data pipelines, making it an invaluable asset for projects requiring the manipulation and analysis of large-scale data.
XGBoost
XGBoost, or eXtreme Gradient Boosting, has carved a niche for itself as a leading machine learning framework for structured data analysis. Praised for its efficiency and performance, XGBoost leverages the gradient boosting algorithm to enhance model accuracy iteratively. Its use of regularisation techniques helps in preventing overfitting, thereby improving the model’s generalisation to new data.
One of the standout features of XGBoost is its parallel processing capability, which, when combined with its sophisticated tree-pruning algorithm, not only speeds up the process, but also simplifies the resulting models for better interpretability and performance. This is also one of the key topics taught in our AI200: Applied Machine Learning course.
Fast.ai
Fast.ai emerges as a powerful machine learning framework for deep learning practitioners, built on the robust foundations of PyTorch. It distinguishes itself with a user-friendly interface and a library that can generate comprehensive training data to enhance model performance.
Fast.ai can be integrated with libraries such as PyTorch to build and train deep learning models and complex machine learning models. However, despite its ease of use, one of its drawbacks is that it may be difficult for beginners to pick up, has limited customisations, and contains many dependencies.
Keras
Keras is a high-level neural networks API, written in Python and capable of running on top of TensorFlow, CNTK, or Theano. Designed with the aim of enabling fast experimentation and prototyping, Keras is user-friendly, modular, and extensible. It’s particularly favoured for its ease of use and simplicity in facilitating quick and easy prototyping of deep learning models.
Keras allows for easy and fast coding, which is accessible to beginners and also sufficiently robust for research professionals to experiment with new ideas. This framework is used widely in industry and academia for a variety of applications ranging from predicting financial trends to developing new drug therapies.
MXNet
MXNet is a powerful, scalable deep learning framework, supported by Amazon Web Services (AWS). It’s designed for both efficiency and flexibility in research prototyping and production deployment, supporting a wide variety of programming languages including Python, R, Scala, and C++.
MXNet is distinguished by its capabilities in handling sparse data, which is essential for handling images and text efficiently. Its dynamic dependency scheduler dynamically determines data dependencies in the computation graph, which optimizes memory usage and computation. The framework also scales almost linearly across multiple GPUs and multiple machines, which is a significant advantage for projects requiring massive computational power.
Caffe
Caffe is a deep learning framework made with expression, speed, and modularity in mind. Developed by the Berkeley Vision and Learning Center (BVLC) and community contributors, Caffe is known for its speed in training convolutional networks. It’s widely used in academic research projects and industry applications, especially those that benefit from its ability to process over 60M images per day with a single NVIDIA K40 GPU.
Caffe provides a clean and modifiable framework, which makes it easy to experiment with new architectures for convolutional and fully connected networks. Its architecture encourages application and innovation in deep learning research by providing the ability to switch between CPU and GPU by setting a single flag to train on a GPU machine and then deploying it to commodity clusters or mobile devices.
Most Popular Machine Learning Libraries
Machine learning libraries play a crucial role in providing pre-written codes and routines that simplify complex tasks. These libraries are collections of functions and methods that allow data scientists and engineers to perform various machine-learning operations without having to write code from scratch. They help in manipulating data, performing mathematical computations, and training machine learning models efficiently. By leveraging these libraries, practitioners can significantly reduce development time and focus more on refining algorithms and experimenting with new models, speeding up the innovation process.
Learn more about some of the popular machine learning libraries below:
pandas
pandas is an open-source data analysis and manipulation library for Python, renowned for its ease of use and performance. It provides flexible data structures, such as DataFrames and Series, which make manipulating data intuitive and efficient.
In the context of machine learning, pandas is primarily used for data preprocessing. It offers powerful tools for cleaning, transforming, and analysing data, which are preliminary yet crucial steps before feeding data into machine learning algorithms. The library simplifies tasks like handling missing data, merging datasets, and filtering columns or rows, which enhances the quality of the data and thereby the reliability of machine learning models built using that data.
Scikit-learn
Scikit-learn is a widely acclaimed library in Python that offers simple and efficient tools for predictive data analysis. It’s built on NumPy, SciPy, and matplotlib, and provides a comprehensive range of well-established machine learning algorithms.
Scikit-learn is celebrated for its extensive collection of algorithms for classification, regression, clustering, and dimensionality reduction, alongside utilities for model selection, evaluation, and preprocessing. The library’s consistent interface across all methods makes it exceptionally user-friendly and highly accessible for newcomers to machine learning. It’s particularly advantageous for prototyping efficient solutions as it helps data scientists quickly implement and evaluate different models.
NumPy
NumPy is a fundamental package for scientific computing in Python. It introduces powerful N-dimensional array objects and provides a suite of functions for working with these arrays.
For machine learning, NumPy serves as one of the most popular libraries, used extensively behind the scenes to handle the numerical operations that libraries like TensorFlow and Scikit-learn depend on. Its ability to perform complex mathematical computations quickly and efficiently makes it indispensable, particularly for tasks involving linear algebra, random number capability, and Fourier transforms, which are common in machine learning applications.
Matplotlib
Matplotlib is a plotting library for Python which provides an object-oriented API for embedding plots into applications. It’s useful for creating static, interactive, and animated visualisations in Python.
In machine learning, Matplotlib plays a crucial role in data exploration and analysis by enabling visualisations of data and models. The ability to plot complex graphs and charts such as line graphs, scatter plots, histograms, and bar charts helps data scientists to understand data trends and patterns, debug aspects of algorithm behaviour, and communicate findings effectively.
TensorFlow
TensorFlow is an end-to-end open-source platform for machine learning designed by Google Brain. It facilitates the creation of complex machine learning models with its flexible and comprehensive toolkit.
TensorFlow is used for both research and production at Google, integrating seamlessly from the research phase of developing algorithms to deployment. Its key features include robust support for deep learning techniques, which involve large neural networks. TensorFlow allows for easy scaling and offers multiple abstraction levels for building and training models, which makes it highly versatile for various machine learning tasks. The library also supports distributed computing, allowing models to be trained on multi-GPU setups or even across clusters of computers, which is ideal for dealing with large and complex datasets.
Most Popular Types of Machine Learning Tools
The machine learning lifecycle is supported by a variety of tools, each serving different purposes from data preparation to model deployment. Below are some of the most used and popular types of tools in machine learning.
Data Preprocessing and Analysis Tools
- Pandas and NumPy are essential for data manipulation, offering powerful functions for transforming raw data into a format suitable for ML applications.
IDEs and Notebooks
- Jupyter and Google Colab provide interactive environments for developing and testing machine learning models, facilitating rapid prototyping and collaboration.
Visualisation Tools
- Matplotlib, Seaborn, and Plotly are crucial for data exploration and visualising model performance, helping to interpret the results of machine learning models.
Model Evaluation and Hyperparameter Tuning Tools
- Tools like GridSearchCV facilitate the fine-tuning of model parameters, optimising model performance.
Deployment and Monitoring Tools
- TensorFlow Serving and MLflow are essential for deploying models into production and monitoring their performance, ensuring models remain effective over time.
Most Popular Machine Learning Tools
The rapid growth of machine learning technology has led to the creation of many tools and among these, some tools stand out for their comprehensive features, ease of use, and strong performance. These tools streamline complex machine learning processes, making sophisticated AI technologies accessible to both novices and experts. Below, we explore some of the most popular machine learning tools that are transforming the way we handle data-driven projects.
Microsoft Azure Machine Learning
Microsoft Azure Machine Learning is a cloud-based platform tailored for building, training, and deploying machine learning models at scale. It provides tools that streamline the entire data science lifecycle, from data prep to model training and deployment.
Azure Machine Learning supports various programming languages and frameworks, offers a collaborative environment, and integrates seamlessly with other Azure services, enhancing its functionality and flexibility. The tool’s ability to manage resources effectively, coupled with built-in governance, allows users to optimise model performance and costs, making it a preferred choice for enterprises looking to scale their machine learning initiatives.
IBM Watson
IBM Watson stands out as a suite of AI services, applications, and tools designed to help organisations predict and shape future outcomes, automate complex processes, and optimise employees’ time.
Watson provides a broad array of machine learning and data analysis capabilities, including natural language processing, computer vision, and prediction capabilities. Its powerful analytics are accessible through an intuitive interface, making advanced data analysis available to non-experts. IBM Watson is particularly known for its robust security features and enterprise-grade solutions that are widely used in industries such as healthcare, finance, and customer service.
Amazon SageMaker
Amazon SageMaker is a fully managed service that provides every developer and data scientist with the ability to build, train, and deploy machine learning models quickly. SageMaker removes the heavy lifting from each step of the machine-learning process to make it easier to develop high-quality models. It provides modular, flexible tools and lets you seamlessly transition from building and training to deployment. With broad framework support, one-click training, and deployment, as well as integrated Jupyter notebooks for easy data exploration, SageMaker is tailored for efficiency and speed.
BigML
BigML offers a consumable, programmable, and scalable machine learning platform that enables easy integration of machine learning capabilities into any business process. Suited for developers, analysts, and data scientists alike, BigML provides a comprehensive suite of machine learning resources, including classification, regression, clustering, anomaly detection, and time series forecasting. BigML focuses on making machine learning approachable with its interactive visualisations and user-friendly interface, which greatly simplify the tasks of designing and deploying models.
Vertex AI
Vertex AI by Google Cloud is designed to accelerate the deployment and maintenance of artificial intelligence models. With Vertex AI, both novice and expert users can implement machine learning operations at scale, utilising Google’s state-of-the-art transfer learning and AutoML technology. This tool integrates various AI capabilities under one unified API, simplifying the process of building and managing AI projects. Vertex AI supports diverse machine learning models, from custom-built to pre-trained options, offering flexibility and power for a wide range of applications, from enterprise-level solutions to innovative startups.
Best Practices in Machine Learning
Effective machine learning model development involves thorough data preparation, careful model selection, and iterative refinement. Staying updated with advancements in machine learning through continuous learning, participating in forums and communities, and engaging with the latest research are also key strategies for success in the field.
Structured Project Organisation
- Adopt a consistent folder structure, naming conventions, and file formats.
- Document and make workflows easily accessible for team collaboration.
Thoughtful ML Tool Selection
- Understand project requirements before choosing machine learning tools and frameworks.
- Consider ease of use, community support, and integration capabilities with existing infrastructure.
Process Automation
- Automate data preprocessing, model training, and deployment processes to enhance efficiency and consistency.
Foster Experimentation and Maintain Experiment Logs
- Encourage trying new algorithms and techniques.
- Use experiment management platforms for tracking and sharing results.
Embrace Organisational Agility
- Stay informed about new machine learning technologies and practices.
- Be adaptable to changing project goals and methodologies.
Ensure Reproducibility
- Implement version control for code and data.
- Use containerisation to maintain consistency across environments.
Rigorous Data Validation
- Conduct thorough data quality checks and validation.
- Use appropriate data-splitting techniques for model training and evaluation.
Monitor and Optimise Resource Usage
- Keep track of and optimise compute, storage, and network resource utilisation to manage expenses.
Assess and Enhance MLOps Maturity
- Regularly evaluate MLOps practices and set improvement goals.
- Continuously refine processes based on feedback and evolving project needs.
Continuous Monitoring and Testing of Machine Learning Models
- Monitor model performance in production and use automated testing for the machine learning pipeline.
- Implement automated responses for detected issues to ensure model accuracy and efficiency.
How to Stay Updated with Machine Learning Advancements
Follow Leading Machine Learning Researchers and Practitioners
- Platforms like Twitter, LinkedIn, and personal blogs are excellent sources for the latest insights and discoveries in machine learning.
Participate in Forums and Communities
- Engage with communities on Reddit (e.g., r/MachineLearning), Stack Overflow, and specialised forums like Cross Validated. These platforms are invaluable for learning from discussions, asking questions, and staying connected with the machine learning community.
Attend Conferences and Workshops
- Events like NeurIPS, ICML, and domain-specific conferences offer opportunities to learn about cutting-edge research, network with professionals, and share your own work.
Continuous Learning
- Enrol in online courses, attend webinars, and read recent papers. Preprint servers like arXiv and journals provide access to the latest research findings.
Implement and Experiment
- Hands-on experience is crucial. Implementing research papers or participating in competitions like those on Kaggle can provide deep insights and practical skills.
Open-Source Contribution
- Contributing to open-source machine learning projects can be a great way to learn, contribute to the community, and collaborate with other machine learning practitioners.
Conclusion
In conclusion, while there is no definitive “best” language for machine learning, each language excels in contexts where it fits best. Hence, the most popular choice of a machine learning language often depends on the specific business problem at hand.
As technology continues to advance, the importance of upskilling and continuous learning in the technological field cannot be overstated. Keeping pace with the latest developments ensures professionals remain competitive and capable of tackling evolving challenges.
For those looking to learn about Python or ML, Heicoders Academy offers comprehensive courses like the A100: Python Programming and Data Visualisation and A200: Applied Machine Learning in Singapore, providing an excellent foundation for beginners and career transitioners alike.
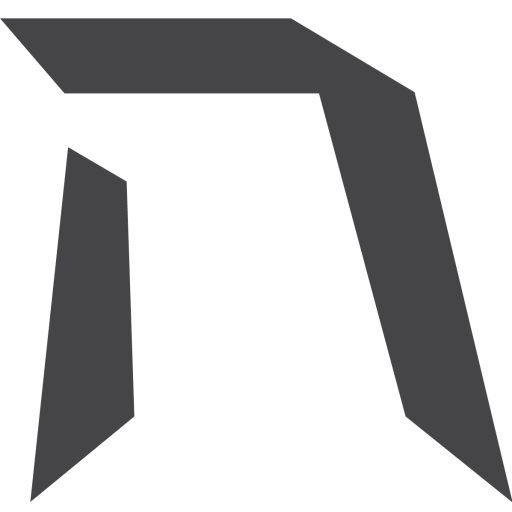
Upskill Today With Heicoders Academy
Secure your spot in our next cohort! Limited seats available.